


Saniya Sood
The integration of AI SDRs into signal-based outbound strategies on LinkedIn represents a paradigm shift in B2B sales development. While the potential benefits are substantial—higher response rates, improved efficiency, and enhanced personalization—implementation is not without significant challenges.
This guide explores the common roadblocks organizations face when deploying AI SDRs for signal-based outbound on LinkedIn and provides practical solutions to overcome them.
The Promise vs. Reality Gap in AI SDR Implementation
Before diving into specific challenges, it's important to understand the disconnect that often exists between expectations and reality when implementing AI SDRs for LinkedIn outbound:
Expectation | Reality | Bridging the Gap |
---|---|---|
Immediate results | 4-8 week ramp-up period | Set realistic timelines and milestones |
Set-it-and-forget-it | Requires ongoing optimization | Establish continuous improvement processes |
Full automation | Human-AI collaboration needed | Define clear roles and handoff points |
Universal personalization | Context-specific personalization | Develop signal-specific frameworks |
Out-of-box performance | Customization required | Invest in proper configuration |
With this reality check in mind, let's examine the specific challenges and their solutions.
Challenge 1: Signal Quality and False Positives
Perhaps the most fundamental challenge in signal-based outbound is distinguishing between genuine buying intent and casual browsing behavior on LinkedIn.
The Problem:
Profile views may represent curiosity rather than buying intent
Content engagement could be professional networking rather than solution research
Job changes might be unrelated to your solution area
Multiple signals from unqualified prospects create noise
When AI SDRs act on low-quality signals, they generate low-quality conversations, wasting resources and potentially damaging your brand.
Solution: Multi-Dimensional Signal Qualification
Implement a comprehensive signal qualification framework:
Signal Strength Scoring
Assign weighted values to different signal types (e.g., pricing page visit = 8, blog engagement = 2)
Consider signal recency (signals within last 24 hours score higher)
Factor in signal duration (time spent engaging)
Track signal frequency (multiple signals from same account)
Signal Correlation Analysis
Look for patterns across multiple signals
Establish minimum threshold scores before triggering outreach
Develop signal clusters that indicate higher intent
Create company-level signal aggregation
Signal Verification Processes
Confirm ICP alignment before acting on signals
Implement role-based signal filtering
Use AI to analyze signal context (e.g., content of comments)
Establish signal blacklist for non-prospects
Valley's multi-dimensional signal qualification stands apart, with proprietary algorithms that significantly reduce false positives while identifying genuine intent signals other platforms might miss.
Challenge 2: Personalization at Scale Without Appearing Intrusive
The second major challenge involves crafting personalized messages based on signals without seeming invasive or "creepy."
The Problem:
Referencing LinkedIn profile views can feel like surveillance
Mentioning specific page visits may violate privacy expectations
Over-personalization can create uncanny valley effect
Generic personalization fails to leverage signal context
When AI SDRs get this balance wrong, prospects feel uncomfortable rather than understood.
Solution: Contextual Personalization Framework
Develop a sophisticated approach to signal-based personalization:
Signal-Appropriate References
Create tiered reference frameworks for different signal types
Use indirect acknowledgment for low-intensity signals
Develop casual reference approaches for medium signals
Implement direct references only for high-intent signals
Content-Based Bridges
Reference related content rather than specific behaviors
Create topic-based outreach rather than behavior-based
Focus on value delivery rather than signal observation
Use indirect language ("I noticed you're interested in..." vs. "I saw you viewed...")
Personalization Balance Matrix
Signal Type | Reference Approach | Example |
---|---|---|
Profile View | Indirect/None | "Given your role in [industry], I thought you might find this valuable..." |
Content Engagement | Topic-Based | "Your comment on the discussion about [topic] was insightful. This related resource might interest you..." |
Website Visit | Solution-Oriented | "Many [role] leaders are exploring solutions for [challenge related to page visited]. I thought you might find this perspective valuable..." |
Multiple High-Intent Signals | Direct but Professional | "I noticed your interest in [topic area] and wanted to share how we've helped similar companies address this challenge..." |
This framework allows for personalization that acknowledges context without crossing privacy boundaries.
Challenge 3: LinkedIn Platform Limitations and Compliance
LinkedIn itself presents several technical and compliance challenges for AI SDR implementation.
The Problem:
LinkedIn's commercial use policy restricts automation
Connection request limits can hamper outreach scale
Message restrictions for non-connections limit reach
Profile view notifications create visibility concerns
Algorithm changes impact signal visibility
When AI SDRs violate LinkedIn policies, accounts can be restricted or banned.
Solution: Platform-Compliant Implementation
Implement a LinkedIn-friendly AI SDR approach:
LinkedIn-Compliant Automation
Maintain human oversight of all connection requests
Implement reasonable daily activity limits
Avoid prohibited scraping activities
Ensure proper authorization for account access
Alternative Signal Leveraging
Use signals to inform email outreach when LinkedIn connection doesn't exist
Develop multi-channel strategies that don't rely solely on LinkedIn
Create content that generates inbound signals rather than just outbound
Implement website visitor identification to complement LinkedIn signals
Risk Mitigation Strategies
Rotate between multiple team accounts rather than using a single account
Implement warming periods for new accounts
Maintain natural activity patterns that mimic human behavior
Develop contingency plans for account restrictions
Challenge 4: Data Integration and Signal Flow
A fourth significant challenge involves integrating LinkedIn signal data with other systems to create a unified view of prospect activity.
The Problem:
LinkedIn signals exist in isolation from other channels
CRM integration limitations create data silos
Signal history often lacks persistence
Cross-channel signal correlation is difficult
Manual data entry creates inconsistencies
Without proper integration, the signal intelligence your AI SDR gathers becomes fragmented and less valuable.
Solution: Unified Signal Intelligence Architecture
Implement a comprehensive data integration strategy:
Technical Integration Framework
Establish bi-directional sync between LinkedIn, AI SDR platform.
Implement webhook-based real-time signal transfer
Create unified prospect profiles that aggregate signals across channels
Develop signal timeline visualization across touchpoints
Signal Taxonomy Standardization
Create consistent signal classification across platforms
Standardize scoring methodology across channels
Implement unified tagging system for signal types
Establish common data dictionaries for signal attributes
Cross-Channel Signal Correlation
Match LinkedIn identities with email and website visitors
Create account-level signal aggregation
Implement buying committee mapping
Establish signal journey tracking across channels
Challenge 5: Human-AI Collaboration and Workflow
The fifth major challenge involves creating effective workflows between AI SDRs and human sales team members.
The Problem:
Unclear handoff points between AI and humans
Sales team resistance to AI collaboration
Inconsistent follow-up on AI-generated opportunities
Misalignment between AI messaging and human conversations
Lack of feedback loop for AI improvement
When these collaboration issues aren't addressed, the potential of AI SDRs goes unrealized.
Solution: Structured Collaboration Framework
Develop a comprehensive human-AI collaboration model:
Clear Role Definition
Establish explicit AI SDR responsibilities vs. human responsibilities
Define specific trigger conditions for human handoffs
Create response templates for common human takeover scenarios
Implement clear ownership transitions
Seamless Handoff Process
Provide complete signal and conversation context during transitions
Create notification systems for required human actions
Implement conversation continuity protocols
Establish SLAs for human response times
Team Adoption Strategy
Provide comprehensive training on signal-based methodology
Create visible success metrics to demonstrate AI SDR value
Implement gradual rollout to build trust
Establish feedback mechanisms for continuous improvement
Feedback Loop Implementation
Create structured process for humans to provide AI feedback
Document successful and unsuccessful conversation patterns
Implement regular review cycles for AI-generated messaging
Establish continuous learning protocols
The most successful implementations balance automation with human expertise:
AI SDR Responsibility | Human Sales Responsibility |
---|---|
Signal monitoring and scoring | High-value opportunity engagement |
Initial personalized outreach | Complex question responses |
Basic question handling | Objection handling and negotiation |
Meeting scheduling | Meeting preparation and execution |
Follow-up sequences | Relationship deepening |
Activity documentation | Strategy adjustment |
Implementation Roadmap
Addressing Challenges Systematically
Overcoming these challenges requires a structured approach:
Phase 1: Foundation (Weeks 1-2)
Develop comprehensive signal taxonomy
Establish integration architecture
Create personalization frameworks
Define human-AI collaboration model
Phase 2: Pilot Implementation (Weeks 3-4)
Deploy with limited account set (100-200)
Implement strict human oversight
Test signal qualification accuracy
Refine personalization approaches
Phase 3: Challenge Resolution (Weeks 5-8)
Address specific issues identified in pilot
Optimize signal scoring and correlation
Refine personalization balance
Enhance human-AI workflows
Phase 4: Scaled Deployment (Weeks 9-12)
Expand to full account coverage
Implement advanced signal intelligence
Reduce human oversight where appropriate
Establish continuous improvement cycles
Valley: Purpose-Built to Overcome Signal-Based AI SDR Challenges
While many platforms offer AI SDR capabilities, Valley was specifically designed to address the challenges of signal-based outbound on LinkedIn. Unlike competitors that added signal features to existing automation tools, Valley built its entire platform around solving these core challenges:
Advanced Signal Intelligence: Valley's proprietary algorithms significantly reduce false positives while identifying genuine intent signals that other platforms might miss.
Contextual Personalization Engine: Valley crafts personalized messages that reference signals appropriately without crossing privacy boundaries, striking the perfect balance between relevance and respect.
LinkedIn-Native Methodology: Valley's approach prioritizes compliance with LinkedIn's policies while maximizing signal utilization, dramatically reducing account risk.
Unified Signal Architecture: Valley provides seamless integration across channels, creating a comprehensive view of prospect engagement that powers more effective outreach.
Collaborative Workflow Design: Valley's platform was built from the ground up to facilitate seamless collaboration between AI and human team members.
Book a demo today.
By recognizing and proactively addressing these common challenges, organizations can successfully implement AI SDRs for signal-based outbound on LinkedIn, transforming their sales development process from volume-based cold outreach to precisely targeted, signal-driven engagement that resonates with prospects and drives business outcomes.
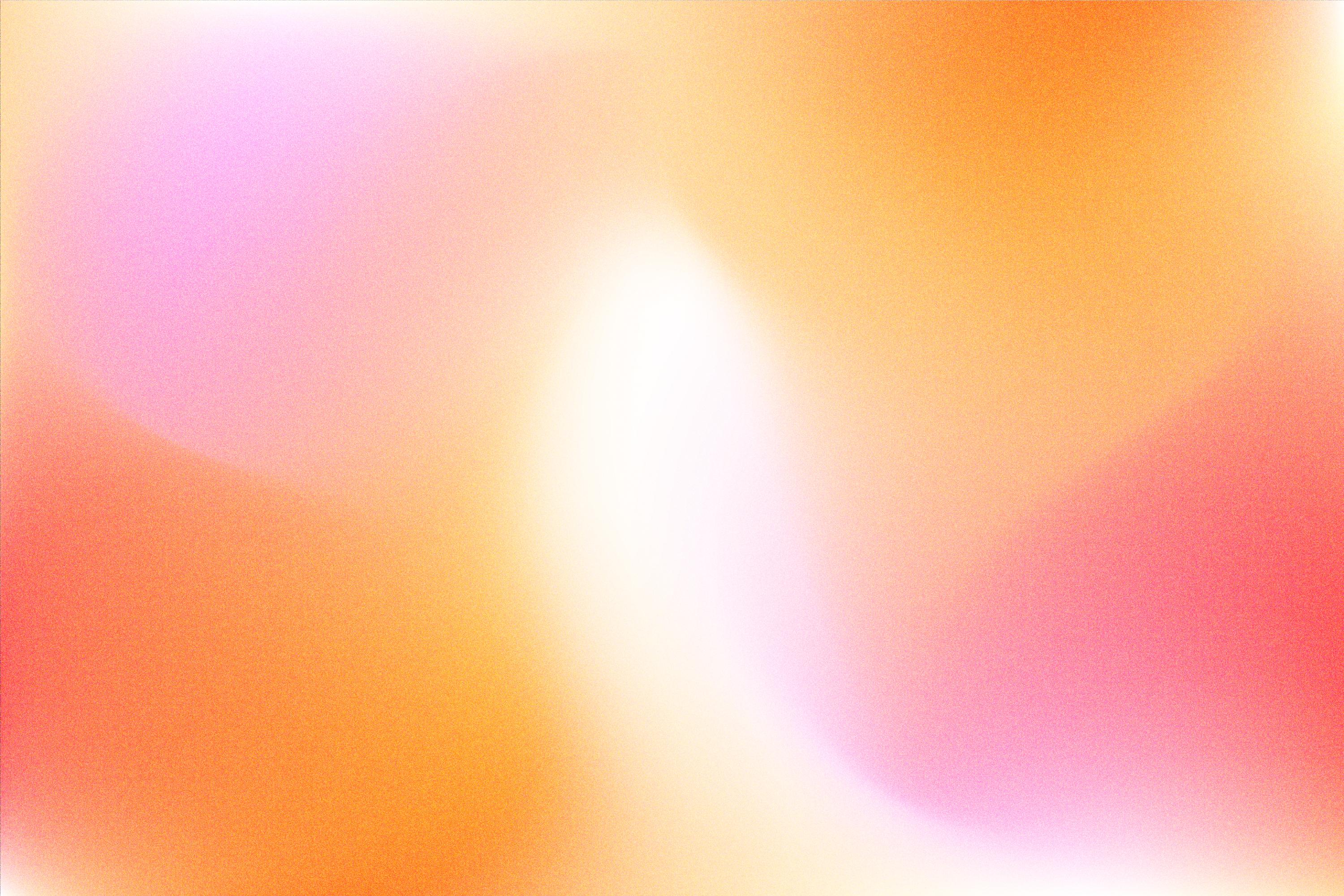
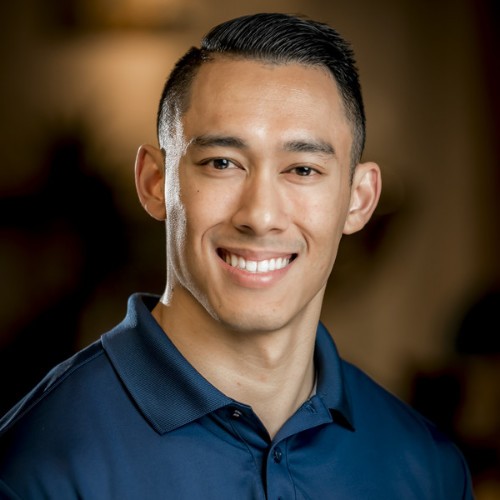