


Saniya Sood
In today's oversaturated B2B landscape, generic outbound messages are increasingly ignored. The evolution from mass outreach to personalized engagement has accelerated dramatically with the emergence of signal-based outbound—a methodology that leverages real-time buying signals to craft hyper-relevant messaging. When powered by AI SDRs, this approach transforms LinkedIn outreach from generic connection requests to context-rich conversations that resonate with prospects.
The Personalization Crisis in Outbound Sales
Before exploring how AI SDRs revolutionize personalization, it's essential to understand the current crisis in outbound engagement:
Statistics | Impact |
---|---|
🔹 21% open rate for standard cold emails | Majority of outreach never gets seen |
🔹 1-3% response rate to generic outreach | Tremendous waste of sales resources |
🔹 98% of prospects delete messages without reading | Opportunity cost of failed connections |
🔹 89% of buyers ignore outreach lacking personalization | Brand damage from poor outreach |
Traditional "personalization" typically means inserting a prospect's name or company—a surface-level approach that today's sophisticated buyers immediately recognize as automated. Signal-based personalization, by contrast, fundamentally transforms this dynamic by leveraging real-time insights about prospect behavior and intent.
Understanding Signal-Based Personalization for LinkedIn Outreach
Signal-based personalization leverages digital behavior patterns—"signals"—that indicate buyer intent or interest. On LinkedIn, these signals include:
Primary LinkedIn Signal Categories
Direct Engagement Signals
Profile views (prospects viewing your team's profiles)
Content engagement (likes, comments, shares)
Connection requests or acceptances
Message responses or inquiries
Professional Milestone Signals
Job changes or promotions
Work anniversaries
Company announcements
New skills or endorsements
Content Affinity Signals
Topic-specific engagement patterns
Hashtag following or usage
Group participation
Content sharing preferences
Extended Network Signals
Connections with competitors
Engagement with partner content
Industry event participation
Mutual connection interactions
Each signal category offers unique personalization opportunities that go far beyond inserting a name or company into a template.
The AI SDR Personalization Framework for LinkedIn
AI SDRs enable sophisticated personalization by analyzing signals and crafting relevant outreach at scale. Here's how leading AI SDR platforms approach signal-based personalization on LinkedIn:
1. Signal Analysis and Interpretation
AI SDRs excel at transforming raw signal data into actionable personalization insights:
Signal Classification
Categorizing signals by type and intent level
Filtering signal noise from meaningful patterns
Correlating signals across time and channels
Identifying signal sequences indicating buying journey stage
Contextual Enrichment
Analyzing content of engaged posts for topic interests
Researching background on professional milestones
Identifying relevant company news and events
Mapping connections to reveal relationship networks
Intent Mapping
Correlating signals with buying journey stages
Identifying solution-specific interest signals
Recognizing research vs. consideration behaviors
Detecting buying committee formation patterns
This sophisticated analysis forms the foundation for truly personalized outreach.
2. Multi-Dimensional Personalization Matrix
Advanced AI SDRs utilize a multi-dimensional approach to personalization:
Personalization Dimension | Example Application | Impact |
---|---|---|
Signal Context | Referencing specific content engagement | Demonstrates relevance and attention |
Professional Context | Acknowledging recent job change | Shows awareness of current situation |
Industry Context | Mentioning sector-specific challenges | Establishes domain expertise |
Solution Alignment | Connecting pain points to capabilities | Creates clear value proposition |
Communication Style | Matching prospect's tone and formality | Builds rapport and comfort |
Engagement History | Referencing previous interactions | Creates conversation continuity |
The most effective AI SDRs combine multiple dimensions to create messages that feel genuinely human and contextually relevant.
3. Personalization Execution Strategies
AI SDRs implement personalization through several strategic approaches:
Signal-Triggered Templates
Contextual Value Narratives
Milestone-Based Outreach
Signal-Centric Questions
These frameworks allow AI SDRs to craft messages that feel human-written while reflecting the specific signals that triggered the outreach.
Signal-Based Personalization Best Practices
Implementing effective signal-based personalization through AI SDRs requires attention to several best practices:
1. Respect the Personalization-Privacy Balance
Personalization must enhance relevance without crossing into invasive territory:
Appropriate Signal References
Acknowledge context without excessive specificity
Reference topics rather than specific actions when possible
Use indirect phrasing for low-intent signals
Respect the "how did you know that?" boundary
Balanced Personalization Guidelines
Signal Type | Appropriate Reference | Too Invasive |
---|---|---|
Profile View | "Given your background in [industry]..." | "I noticed you viewed my profile yesterday at 2:35pm..." |
Content Engagement | "Your insights on [topic] caught my attention..." | "I saw you spent 45 seconds reading my post before commenting..." |
Website Visit | "Many [role] leaders are exploring solutions for [challenge]..." | "I noticed you visited our pricing page three times this week..." |
Job Change | "Congratulations on your recent move to [company]..." | "I see you left [previous company] after only 8 months..." |
Maintaining this balance ensures personalization enhances rather than damages relationship building.
2. Implement Progressive Personalization
Rather than frontloading all personalization into initial outreach, implement a progressive approach:
Tiered Personalization Strategy
Outreach Stage | Personalization Focus | Example |
---|---|---|
Initial Connection | General context and relevant insight | "Given your focus on [industry], I thought this perspective on [trend] might be valuable..." |
First Follow-Up | Signal-based specific relevance | "Since you're interested in [topic from engagement], I wanted to share this specific approach..." |
Subsequent Engagement | Cumulative context and relationship | "Building on our conversation about [previous topic], this new development in [related area] seems relevant to your situation..." |
Meeting Request | Comprehensive value narrative | "Based on your interest in [topic], challenges with [pain point], and goals for [objective], a brief conversation about our approach to [solution area] could be valuable..." |
This progressive approach builds relationship depth while avoiding overwhelming initial outreach.
3. Balance Automation with Human Oversight
The most effective personalization combines AI efficiency with human judgment:
Tiered Review Framework
Account Tier | Personalization Approach | Review Process |
---|---|---|
Strategic Accounts | Deep multi-signal personalization | Human review of all messages |
Target Accounts | Signal-based contextual personalization | Review of initial outreach only |
Scale Accounts | Template with signal-based variables | Spot-checking and exception handling |
This balanced approach ensures personalization quality while maintaining efficiency.
4. Test and Refine Personalization Approaches
Implement systematic testing to optimize personalization effectiveness:
Structured Testing Framework
A/B test different reference styles for similar signals
Compare response rates across personalization dimensions
Evaluate depth vs. breadth personalization approaches
Test tone and formality variations by prospect type
Learning Loop Implementation
Document successful personalization patterns
Identify triggers for negative responses
Develop signal-specific best practices
Create personalization guidelines by industry and role
This commitment to optimization ensures continuous improvement in personalization effectiveness.
Real-World Impact: The ROI of Signal-Based Personalization
Organizations implementing AI SDR-driven signal-based personalization on LinkedIn see dramatic performance improvements:
Metric | Traditional Outreach | Signal-Based Personalization | Improvement Factor |
---|---|---|---|
Connection Acceptance | 15-25% | 45-65% | 2-3x |
Response Rate | 1-3% | 30-45% | 10-30x |
Meeting Conversion | 10-15% | 25-40% | 2-4x |
Sales Cycle | 90+ days | 45-60 days | 33-50% reduction |
Customer Feedback | "Generic" | "Understands my needs" | Qualitative improvement |
These improvements stem from the fundamental shift from generic interruption to contextually relevant conversation that signal-based personalization enables.
Valley Edge
Signal-Based Personalization
Valley has pioneered signal-based personalization for LinkedIn outreach, offering capabilities that significantly outperform other AI SDR platforms:
Unique Valley Personalization Capabilities
Proprietary Signal Intelligence
Advanced detection of subtle buying signals others miss
Multi-channel signal correlation for comprehensive context
Behavior pattern recognition across the buying journey
Signal strength scoring based on conversion likelihood
Contextual Research Engine
Automated background research on signal-generating prospects
Industry-specific challenge mapping
Role-based pain point identification
Company news and event monitoring
Dynamic Message Generation
Signal-specific messaging frameworks
Multi-dimensional personalization matrix
Tone and style matching to prospect communication patterns
Progressive personalization sequencing
These capabilities translate to unmatched personalization effectiveness, with Valley customers reporting 45%+ acceptance rates, 32%+ response rates, and 4-10 booked meetings per month per seat—results that far exceed industry averages for traditional outbound approaches.
Getting Started with Signal-Based Personalization
To implement AI SDR-driven signal-based personalization on LinkedIn:
Establish signal monitoring infrastructure including LinkedIn Sales Navigator, website visitor identification, and content engagement tracking
Develop a comprehensive signal taxonomy defining the signals your team will monitor and how they'll be interpreted
Create signal-specific messaging frameworks for different signal types and combinations
Implement appropriate AI SDR technology capable of detecting, interpreting, and responding to signals
Establish continuous improvement processes to refine personalization based on results
By following these steps and leveraging the right AI SDR platform, sales teams can transform their LinkedIn outreach from generic template-filling to genuinely personalized conversations that engage prospects and drive business results.
Valley's purpose-built platform for signal-based personalization offers the most direct path to achieving these outcomes, combining sophisticated signal intelligence with advanced personalization capabilities to create outreach that truly resonates with prospects at precisely the right moment in their buying journey.
Book a demo today.
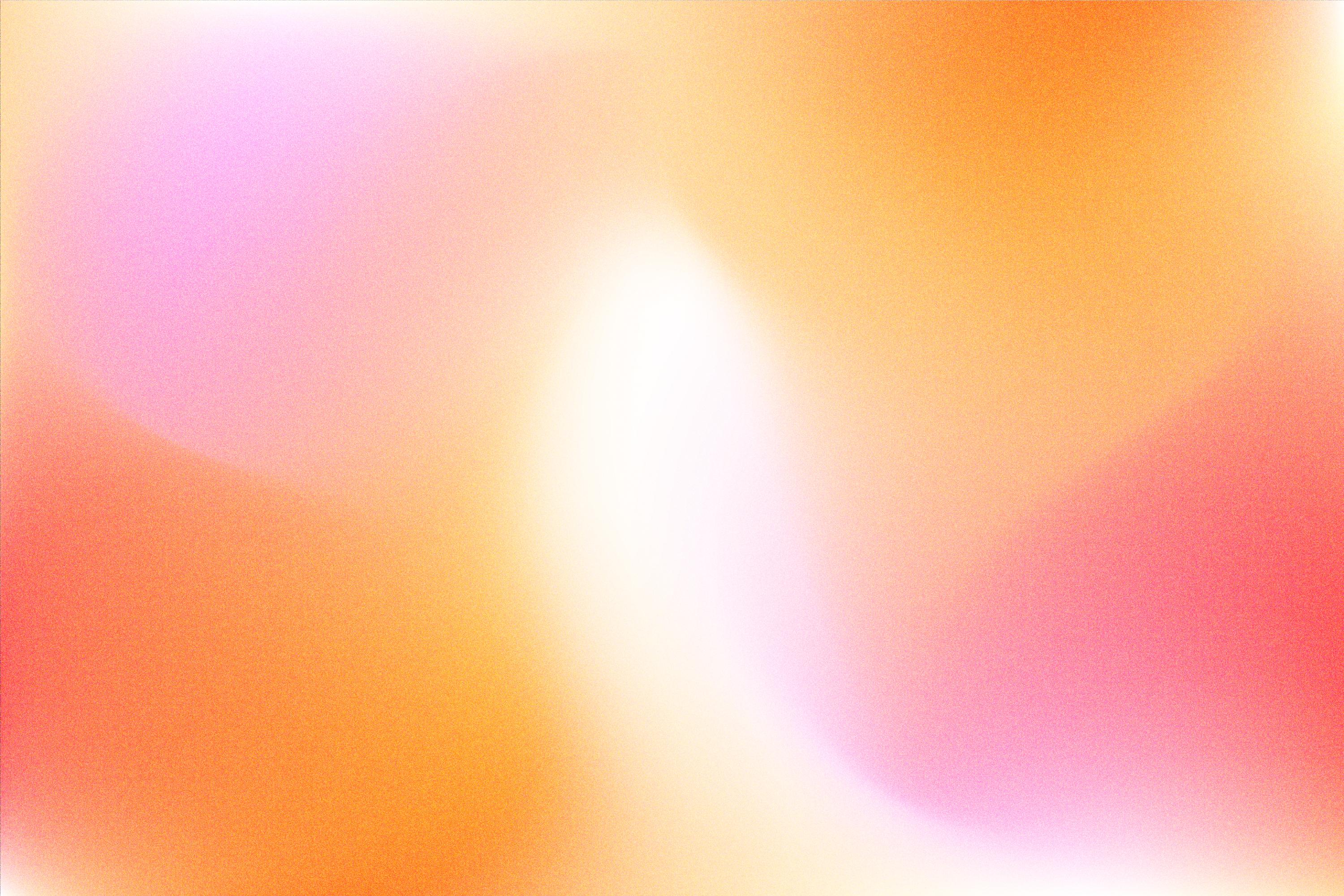
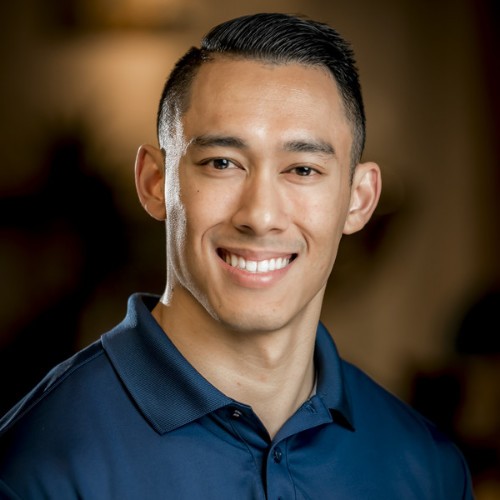