The Evolution of Outbound
Generic outbound approaches has become increasingly ineffective. With response rates plummeting below 1% for traditional cold outreach, leading organizations are turning to sophisticated data-driven strategies to revolutionize their outbound sales approach. This comprehensive playbook explores how leveraging data transforms customer segmentation and targeting, ultimately driving higher conversion rates, improved sales efficiency, and accelerated revenue growth.
From Mass Outreach to Precision Targeting
Outbound sales has undergone a fundamental transformation over the past decade. To understand the power of data-driven approaches, it's essential to recognize how dramatically the landscape has changed.
The Traditional Outbound Approach vs. Data-Driven Methodology
Aspect | Traditional Outbound | Data-Driven Outbound |
---|---|---|
Targeting Basis | Static lists, basic firmographics (industry, company size) | Multi-dimensional data including intent signals, technographics, engagement patterns |
Segmentation Depth | Broad categories with limited variables | Highly granular micro-segments with dynamic composition |
Personalization Level | Surface-level personalization (name, company) | Deep personalization based on specific pain points, behaviors, and needs |
Timing Strategy | Calendar-driven campaigns | Behavioral and intent-triggered outreach |
Lead Prioritization | Often alphabetical or chronological | Sophisticated scoring algorithms identifying high-value opportunities |
Performance Measurement | Basic activity metrics (calls made, emails sent) | Comprehensive conversion analytics tied to revenue impact |
Typical Response Rates | 0.5-2% | 15-35% for high-intent segments |
The Data Foundation: Essential Data Types for Effective Segmentation
Before exploring how data transforms segmentation, it's critical to understand the key data categories that power sophisticated targeting. Each data type provides unique insights that, when combined, create a comprehensive view of potential customers.
Core Data Categories for B2B Segmentation
1. Firmographic Data
Definition: Basic company information that helps identify organizational fit. Examples: Industry, company size, annual revenue, geographic location, growth stage Segmentation Application: Creates foundation for Ideal Customer Profile (ICP) alignment
2. Technographic Data
Definition: Information about the technology stack and tools a company uses. Examples: CRM systems, marketing automation platforms, tech infrastructure, recent implementations Segmentation Application: Identifies technical compatibility and potential integration points
3. Intent Data
Definition: Signals indicating active research or buying interest in solutions like yours. Examples: Content consumption patterns, search behavior, competitor research, event attendance Segmentation Application: Reveals timing and readiness to engage in sales conversations
4. Engagement Data
Definition: How prospects interact with your brand across channels. Examples: Website visits, email opens, content downloads, webinar attendance, social engagement Segmentation Application: Shows level of awareness and interest in specific solutions
5. Historical Performance Data
Definition: Past results from similar prospects and campaigns. Examples: Conversion rates by segment, sales cycle length, win rates, average deal size Segmentation Application: Predicts likely outcomes and optimal approaches for similar prospects
Advanced Data-Driven Segmentation Strategies
With these data foundations in place, organizations can implement sophisticated segmentation strategies that dramatically improve targeting precision. Here's how leading companies leverage data for advanced segmentation:
1. Multi-Dimensional Segmentation Models
Rather than relying on simple one or two-variable segments, data-driven outbound enables complex segmentation based on multiple factors simultaneously.
Implementation Framework:
Where each component is scored on a 0-100 scale based on alignment with ideal parameters.
Example in Action: Instead of simply targeting "Manufacturing companies with 500+ employees," a data-driven approach might target "Manufacturing companies with 500+ employees using SAP systems, actively researching automation solutions in the past 30 days, and engaging with competitor content."
This multi-dimensional approach creates highly specific segments with vastly improved conversion potential.
2. Intent-Based Segmentation
Intent data has transformed how organizations identify and prioritize prospects by revealing which companies are actively in-market for solutions.
Types of Intent Signals:
Intent Type | Data Source | Signal Strength | Action Timeframe |
---|---|---|---|
Direct Intent | Demo requests, pricing page visits | Very High | Immediate (24-48 hours) |
Solution Research | Product comparison content, feature research | High | Short-term (3-7 days) |
Problem Research | Educational content, topic exploration | Medium | Medium-term (1-3 weeks) |
Competitive Engagement | Competitor research, comparison resources | High | Short-term (3-7 days) |
Event-Triggered Intent | Funding announcements, leadership changes | Medium-High | Short-term (1-2 weeks) |
Implementation Best Practices:
Establish intent scoring models that weight different signals based on conversion correlation
Create time-decay factors that reduce signal strength over time
Combine first-party and third-party intent data for comprehensive coverage
Develop intent threshold triggers for automated segmentation
3. Behavioral Segmentation
By analyzing how prospects interact with your brand across channels, behavioral segmentation identifies patterns that indicate interest level, specific needs, and readiness to engage.
Key Behavioral Segments:
Active Researchers: Multiple content interactions across related topics
Product Evaluators: High engagement with technical content and specifications
Price Sensitive: Focus on pricing and ROI content
Competitive Switchers: Engagement with competitor comparison content
Early Stage Explorers: Broad engagement with educational content
Activation Approach: For each behavioral segment, develop specialized messaging tracks that address their specific stage and interests. For example, "Product Evaluators" should receive detailed technical information and case studies, while "Price Sensitive" segments benefit from ROI calculators and value-driven messaging.
4. Predictive Segmentation
The most advanced data-driven organizations use historical performance data to develop predictive models that identify prospects most likely to convert before they even show explicit interest.
Predictive Factors to Analyze:
Conversion patterns from similar companies
Engagement sequences that preceded past purchases
Firmographic and technographic traits of best customers
Optimal timing patterns for different segments
Implementation Steps:
Analyze past wins to identify common characteristics
Develop propensity models that score prospects on likelihood to convert
Create lookalike segments based on your most successful customers
Implement automated scoring and segmentation based on predictive factors
Transforming Targeting
Through Data-Driven Insights
With sophisticated segmentation in place, data-driven outbound enables precision targeting that dramatically improves efficiency and effectiveness.
1. Prioritization Frameworks
Not all prospects deserve equal attention. Data-driven prioritization ensures resources focus on high-value opportunities.
Sample Prioritization Framework:
Priority Level | Criteria | Contact Strategy | Follow-up Cadence |
---|---|---|---|
Tier 1 (Very High) | High intent + High fit + Recent engagement | Direct phone + Personalized email | Accelerated (2-3 touches in first week) |
Tier 2 (High) | Medium-high intent + High fit | Personalized email sequence | Standard (5-7 touches over 2-3 weeks) |
Tier 3 (Medium) | Low intent + High fit OR High intent + Medium fit | Semi-personalized email sequence | Extended (5-7 touches over 3-4 weeks) |
Tier 4 (Low) | Low intent + Medium fit | Automated nurture | Maintenance (monthly touchpoints) |
2. Channel Optimization
Data analysis reveals which channels work best for different segments, enabling targeted multi-channel approaches.
Channel Effectiveness by Segment:
Segment Characteristic | Most Effective Primary Channel | Supporting Channels | Avoid |
---|---|---|---|
C-Suite Executives | Direct phone + Executive networking | LinkedIn InMail, Referrals | Mass email campaigns |
Technical Decision Makers | Personalized email with technical content | LinkedIn engagement, Virtual events | Cold calling |
Mid-level Managers | LinkedIn + Email | Direct phone, Webinars | Direct mail |
Small Business Owners | Direct phone | Email, LinkedIn | Complex multi-touch sequences |
3. Message Personalization at Scale
Data-driven segmentation enables highly personalized messaging without sacrificing efficiency.
Personalization Matrix:
Data Point | Personalization Application | Example |
---|---|---|
Intent Signals | Address specific research interests | "I noticed you've been researching automated appointment scheduling solutions..." |
Technology Stack | Highlight relevant integrations | "As a HubSpot user, you'll appreciate how our solution seamlessly integrates with your existing workflow..." |
Industry Challenges | Reference specific pain points | "Manufacturing companies like yours are facing increasing pressure to optimize production efficiency..." |
Company Events | Acknowledge recent developments | "Congratulations on your recent funding round. As you scale operations, our solution can help..." |
Engagement History | Reference previous interactions | "I saw you downloaded our guide on supply chain optimization..." |
Implementation Approach: Create modular content blocks for each personalization category that can be dynamically assembled based on prospect data. This enables personalization at scale while maintaining message quality and relevance.
4. Timing Optimization
Data analysis reveals optimal timing patterns for different segments and individuals.
Timing Factors to Analyze:
Day of week and time of day engagement patterns
Response latency after specific triggers
Seasonal variations in responsiveness
Industry-specific timing considerations (fiscal year, budget cycles)
Implementation Best Practices:
Develop timing models based on historical engagement data
Identify optimal send windows for different segments
Create trigger-based timing rules for intent signals
Implement automated scheduling based on individual engagement patterns
As B2B buying processes become increasingly complex, data-driven segmentation and targeting will be essential capabilities for high-performing sales organizations. By leveraging comprehensive data insights to create precise segments and personalized targeting strategies, companies can dramatically improve conversion rates, accelerate sales cycles, and maximize resource efficiency.
The most successful organizations will be those that continuously refine their approach based on performance data, implementing increasingly sophisticated segmentation models while maintaining a relentless focus on delivering relevance and value to prospects.
Valley's platform helps B2B companies implement data-driven outbound strategies through its comprehensive signal-based solution. By identifying website visitors, tracking intent signals, and automating personalized outreach, Book a demo & see how Valley enables sales teams to focus on closing deals with the most promising prospects while dramatically improving targeting precision and conversion rates.
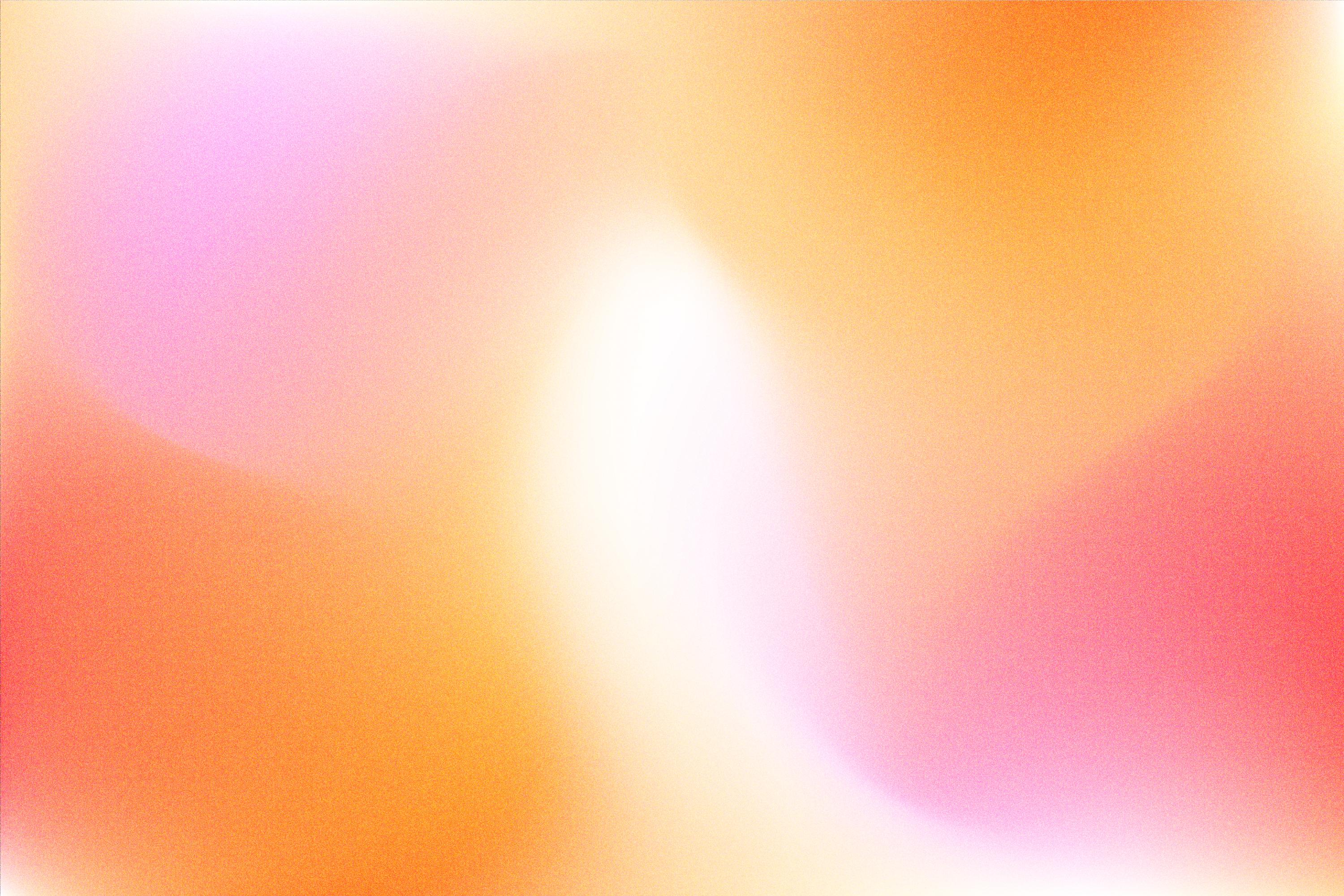
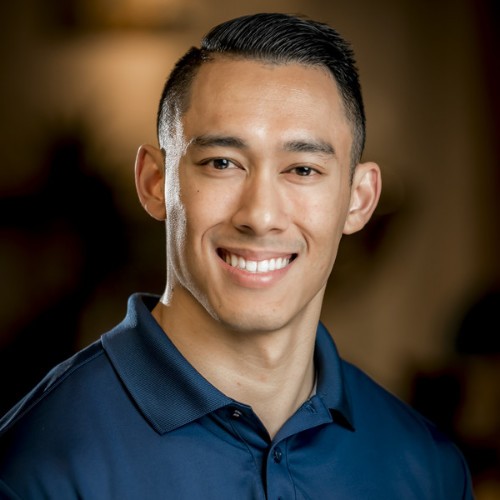