The Signal-Based Foundation:
The integration of AI SDR technology with LinkedIn has revolutionized outbound sales, moving teams beyond generic cold outreach toward strategic, signal-driven engagement. However, successful implementation requires more than simply deploying technology—it demands a thoughtful approach that combines advanced tools with strategic methodology.
This guide explores proven best practices for integrating AI SDRs with LinkedIn to create a signal-based outbound strategy that generates meaningful conversations and measurable results.
Understanding the New Framework
Before diving into integration best practices, it's crucial to understand how signal-based outbound differs from traditional approaches:
Element | Traditional Outbound | Signal-Based Outbound |
---|---|---|
Trigger | Sales rep calendar | Prospect behavior signals |
Timing | Predetermined sequence | Real-time response to intent |
Targeting | Static ICP criteria | Dynamic behavior patterns |
Personalization | Template + variables | Signal-specific context |
Value Proposition | Generic solution benefits | Tailored to observed needs |
Messaging Volume | High (quantity-focused) | Strategic (timing-focused) |
With this framework in mind, let's explore how to effectively integrate AI SDRs with LinkedIn for maximum impact.
Best Practice 1: Establish a Comprehensive Signal Taxonomy
The foundation of effective signal-based outbound is a well-defined signal taxonomy—a systematic classification of the buying signals your AI SDR will monitor and act upon.
LinkedIn Signal Categories to Monitor
Direct Engagement Signals
Profile views from target accounts
Connection requests
InMail or message responses
Content engagement (comments, likes, shares)
Professional Milestone Signals
Job changes
Work anniversaries
Company expansions/acquisitions
New responsibilities
Content Interaction Signals
Engagement with specific content topics
Pattern of content consumption
Depth of engagement (view vs. comment)
Sharing of industry-related content
Digital Body Language
Frequency of profile visits
Time spent on content
Sequence of engagement activities
Cross-platform engagement patterns
For each signal type, define:
Intent score (1-10)
Response urgency (immediate, same-day, within week)
Appropriate message type
Escalation path
This taxonomy becomes the intelligence framework your AI SDR will leverage to prioritize outreach.
Best Practice 2: Implement Multi-Level LinkedIn Integration
Effective AI SDR integration requires connecting with LinkedIn at multiple levels:
Technical Integration Elements
Sales Navigator API Connection
Configure your AI SDR to access Sales Navigator data
Set up saved searches aligned with your ICP
Establish real-time alert monitoring
Implement account and lead list synchronization
Website-to-LinkedIn Identification
Install website visitor tracking with LinkedIn profile mapping
Create signal workflows triggered by specific page visits
Establish threshold rules for engagement scoring
Implement privacy-compliant data handling
CRM Synchronization
Bi-directional data flow between LinkedIn, AI SDR, and CRM
Unified activity timeline across platforms
Consolidated engagement history
Automated signal documentation
Content Engagement Tracking
Monitor post analytics for engagement signals
Track content topic affinity by prospect
Identify content sharing patterns
Map engagement to buying journey stages
This multi-faceted integration ensures no valuable signal is missed and all data flows seamlessly between systems.
Best Practice 3: Develop Signal-Specific Messaging Frameworks
Generic messages fail to capitalize on the power of signals. Instead, create tailored messaging frameworks for each signal type:
Signal-Specific Messaging Examples
For Website Visit Signals
For Content Engagement Signals
For Job Change Signals
For Multiple Signal Patterns
Equip your AI SDR with these frameworks, but ensure they maintain enough flexibility to incorporate specific signal details and prospect context.
Best Practice 4: Balance Automation with Human Oversight
While AI SDRs excel at scaling signal-based outreach, strategic human oversight remains essential:
Effective Human-AI Collaboration Model
Fully Automated
Initial signal detection and scoring
Routine engagement monitoring
Basic personalization and outreach
Standard follow-up sequences
Human Review Required
High-value account outreach
Complex multi-stakeholder situations
Nuanced response handling
Strategic account planning
Human-AI Handoff Triggers
Specific response types (e.g., detailed questions)
Engagement threshold reached
Multiple stakeholder involvement
Advanced stage signals
Establish clear protocols for when the AI SDR should operate autonomously and when human sales reps should take over, ensuring the right balance between scale and personalization.
Best Practice 5: Implement Continuous Learning Loops
Signal-based outbound is not a set-it-and-forget-it approach. It requires ongoing refinement:
Learning Loop Components
Signal Effectiveness Analysis
Track which signal types generate highest response rates
Identify signal combinations that indicate higher intent
Monitor signal-to-meeting conversion rates
Analyze signal patterns by industry and role
Message Performance Optimization
Test multiple message variations for each signal type
Analyze response rates by message structure and length
Optimize subject lines for different signals
Refine personalization approaches based on results
Signal Scoring Adjustment
Regularly calibrate signal importance weightings
Update urgency classifications based on conversion data
Refine signal correlation models
Adjust threshold triggers for different account tiers
AI SDR Capability Enhancement
Expand signal types being monitored
Improve personalization algorithms
Refine response handling capabilities
Enhance multi-channel coordination
This commitment to continuous improvement ensures your signal-based approach evolves with changing market dynamics and prospect behaviors.
Implementation Roadmap
A Phased Approach
Implementing AI SDR integration with LinkedIn is best approached in phases:
Phase 1: Foundation (Weeks 1-2)
Establish signal taxonomy and scoring framework
Configure technical integrations
Define initial message templates
Set up basic automation workflows
Phase 2: Pilot (Weeks 3-4)
Launch with limited account set (100-200 accounts)
Monitor closely for signal detection accuracy
Test message variations
Refine handoff processes
Phase 3: Optimization (Weeks 5-8)
Analyze initial performance data
Adjust signal prioritization based on results
Refine messaging frameworks
Optimize human-AI collaboration model
Phase 4: Scale (Weeks 9-12)
Expand to full target account list
Implement advanced signal correlation
Develop industry-specific approaches
Establish ongoing optimization processes
This measured approach ensures proper foundation-building before scaling your signal-based efforts.
Valley: The Signal-First AI SDR for LinkedIn Integration
Valley has pioneered signal-based outbound on LinkedIn, offering a purpose-built platform that seamlessly integrates with the professional network. Unlike competitors that retrofitted existing automation tools with signal capabilities, Valley's platform was designed from the ground up for signal detection, interpretation, and response.
Key differentiators include:
Proprietary signal correlation engine that connects LinkedIn activity with website behavior and email engagement
Advanced LinkedIn profile analysis for deeper personalization beyond surface-level variables
Sophisticated intent scoring based on signal patterns specific to your industry and solution
Continuous learning algorithms that refine signal interpretation based on results
By combining these best practices with Valley's purpose-built platform, sales teams can transform LinkedIn from a simple networking tool into a powerful signal-based outbound engine that drives meaningful conversations with prospects at precisely the right moment in their buying journey- Book a demo
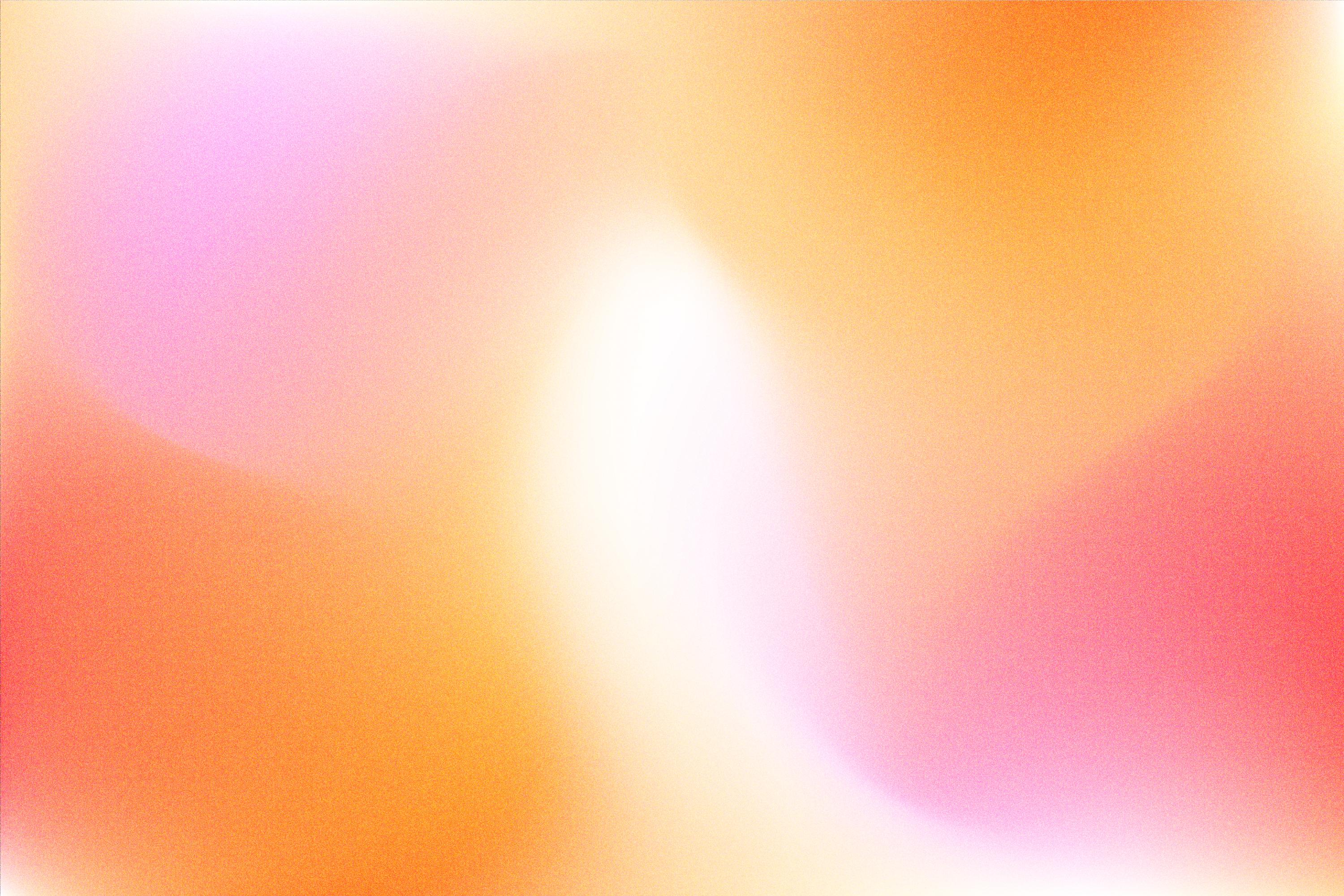
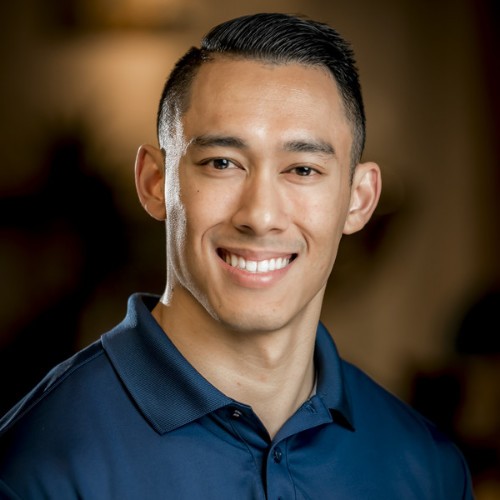